Here are a couple things that add to our class discussion from Thursday.
First, @AdBaum, a Ball State journalism graphics student, posted his storyboards for a graphic he created as part of @BSUattheGames.
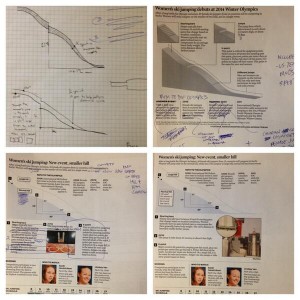
It is a great example of the way the design process works: start with a simple design, move to prototyping, edit, edit more, finish.
The second thing is related to the data plan/story update of Steele and Zoe. If you remember they want to do something about the minimum wage, and they were thinking about linking it to poverty. I told them to try that, but also I suggested they might want to look for something smaller that might be more directly affected by variation in the minimum wage.
Poverty is a complex concept and is influence by a number of variables. Raising the minimum wage could definitely result in a change in the poverty rate, but it is hard to parse out the effect of raising the minimum wage from the effects of the other variables.

For example, if minimum wage goes up and a second variable goes down, we would see no difference in the poverty rate. If the number of the variables affecting poverty isn’t just three (like above), but is 100′s it would be nearly impossible to see the the unique effect of any single variable. Therefore, we need to see if we can find an outcome variable, which is more directly related to raising the minimum wage.
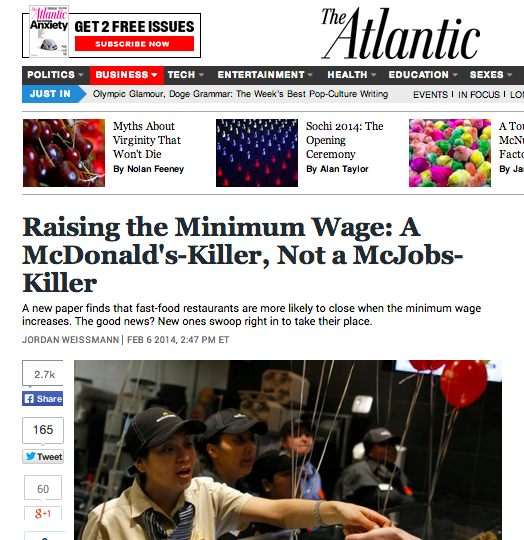
The Atlantic published a story on Thursday that did exactly this. Their article, based on a piece of academic research, argues that raising the minimum wage results in cheap fast food restaurants, such as McDonalds, closing and being replaced by more expensive fast food restaurants, like Five Guys. The article explains:
All of this raises an interesting question: Why would more fast-food spots suddenly start opening right after wages rise? The study’s theory, essentially, is that it’s tough to teach an old McDonald’s new management tricks. Brand new restaurants can be flexible about their business model. But once set, that model can be hard to revamp. So if management is used to paying its cashiers and fry cooks $7.25 an hour, it might never figure out how to clear a profit while paying them $9. A new burger joint, on the other hand, can set itself up to earn money immediately (and take advantage of the hole in the market left by the failed competition).
This article is a great example of two things. First, it show us how we should look for smaller, micro-level effects of policy changes. Second, it shows how to use academic research to discuss social problems. We could easily turn this into more of a “data journalism” story (i.e., something that could fulfill the requirements of this class) by visualizing the academic researchers’ results.